When people explain something to each other, they often monitor signals of incomprehension from the other party to adapt their explanation accordingly. Scientists in a new subproject at the Transregional Collaborative Research Centre ‘Constructing Explainability’ (TRR 318) want to enable this behaviour in machines. But the approach has its pitfalls when it comes to interactions between humans and machines. Three questions for the project leader, Junior Professor Dr Hanna Drimalla from Bielefeld University’s Faculty of Technology:
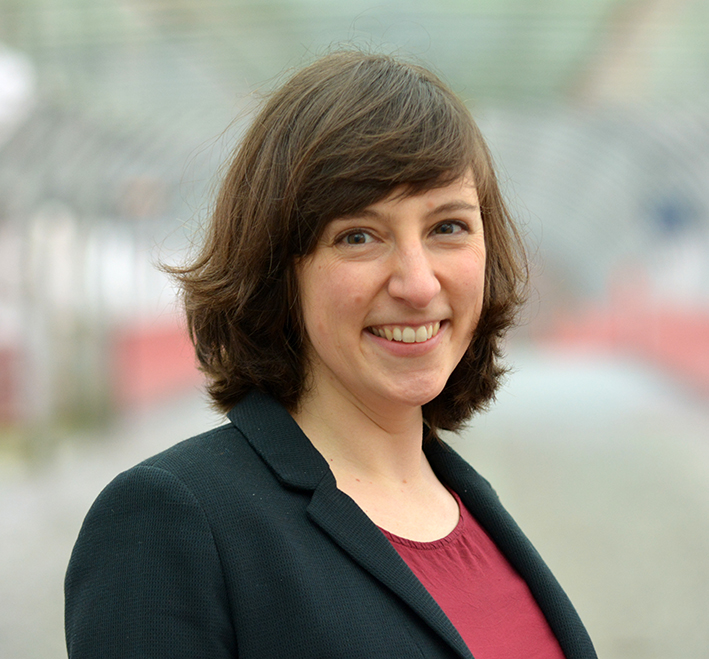
© Bielefeld University
Transregio’s research builds on the fact that understanding is constructed jointly. To what extent does this also apply to systems equipped with artificial intelligence (AI)?
Hanna Drimalla: Currently, there is a lot of research on explainable artificial intelligence, or XAI for short. This involves, for example, a machine explaining something to a human being—such as why it has decided to do something in particular. However, there are always two sides to an explanation, and this is an idea that has still hardly been taken into account in XAI research. If an explanation process is to succeed, it requires feedback loops. A human or a machine explains something while simultaneously monitoring whether the other party understands the explanation and how they react to it. Then, this information is used to adapt the explanation immediately within the conversation. Hence, when machines provide explanations, they need to generate them together with their users.
However, people differ greatly in the ways they react. Couldn’t that mean that the AI models controlling the machines won’t work equally well for all people?
Hanna Drimalla: Exactly. We are seeking a solution to precisely this challenge: that people differ from each another. Some people communicate their misunderstanding by frowning, others avert their gaze or change their facial expressions. A model that always interprets the same signals as understanding or misunderstanding is no use. You can see how problematic this is by looking at people who have a social interaction disorder. People with autism, for example, find it difficult to maintain eye contact. People suffering from depression or social anxiety, in turn, show different social signals. And overall, people behave differently depending on the situation. Someone who is stressed when entering conversation sends different signals to someone who is relaxed. Those who are in a relationship based on power also react differently: People facing an authority person are more likely to mirror that person’s emotional expression.
How do you plan to incorporate these different requirements into your machine models?
Hanna Drimalla: Constructing a separate model of social signals for every psychiatric condition and every situation would be an endless task. Instead, we want to enable each individual to construct the understanding process together with the machine. For example, our models use the automatic recognition of facial expression, gaze behaviour, and voice pitch from video data. We are also working on incorporating data such as heartbeat, which can be detected from red pixel values on the face—in other words, psychophysiological signals. Our goal is to have XAI models that give direct feedback to users on why the machine has recognized understanding or lack of understanding in them. For example, the machine gives the feedback: I understand your frown as indicating that you did not understand something. Then the user can respond by saying that her frown is just due to concentration and the model can realign itself to that. This is the way to make this comprehension monitoring inclusive and fair. It should work for everyone and in every situation.