Should a bank grant someone a loan? Where should police officers be to prevent a crime? And is surgery or conservative treatment recommended for a brain tumour? Artificial intelligence can often provide reliable answers to such questions. However, it is not always clear how the system arrives at its result. Researchers from Bielefeld and Paderborn universities are trying to make such results explainable in a subproject of the Collaborative Research Centre and Transregio ‘Constructing Explainability’ (SFB/TRR 318).
Even if artificial intelligence (AI) frequently provides surprisingly reliable answers, its users tend not to trust its recommendations blindly. They want to understand how an AI reached its conclusion. However, simple explanations are rare: ‘Explaining often involves elucidating complex relationships and interactions between many variables,’ says Professor Dr Axel-Cyrille Ngonga Ngomo from the Department of Computer Science at Paderborn University. Together with Professor Dr Philipp Cimiano from Bielefeld University’s CITEC research institute and Professor Dr Elena Esposito from Bielefeld University’s Faculty of Sociology, he is exploring the foundations of dialogue systems for AI that need to explain their answers to humans.
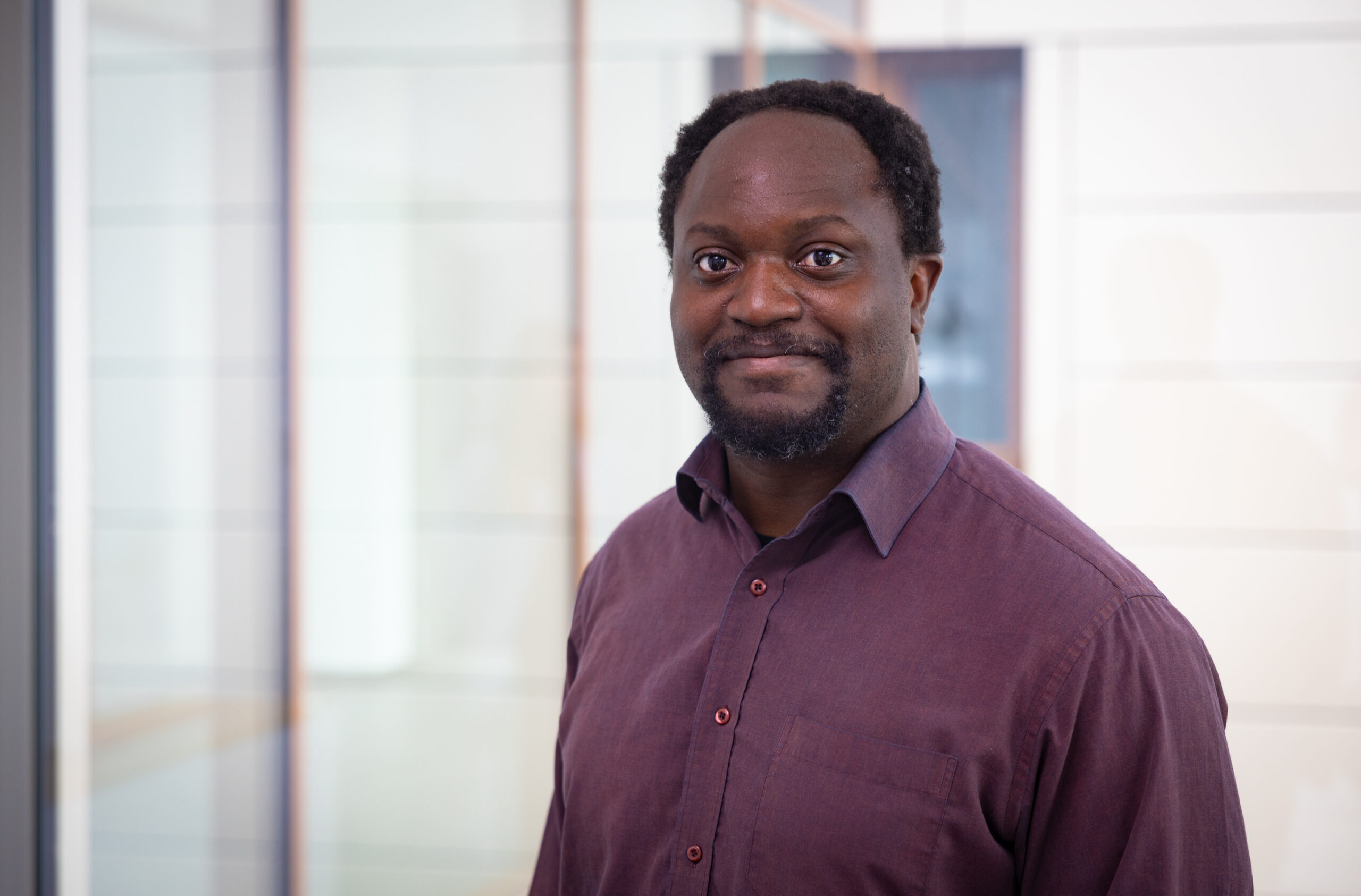
© Bielefeld University/Mike-Dennis Müller
AI-generated explanations require specialist knowledge
One of the challenges here, for example, is that the reason why a person is classified as uncreditworthy does not have to be based on the sum of the individual variables, but can also result from their interaction. How can a machine provide a meaningful explanation of how it arrived at its result in such a case? Such explanations often require a lot of knowledge about how an AI works—and very often they would be far too complex even for AI experts if it were necessary to understand the entire process.
So how can we better construct understanding? ‘One way is to work with a counterfactual approach instead of in-depth explanations,’ says Ngonga. That means explaining something about the opposite of an outcome. In this case, a chatbot would make it clear that it would have made a different decision if a few crucial details had been different. For example, in the case of the loan, it would have been granted if the person needing the money was not currently already paying off a car.
New system to take into account earlier communication with questioners
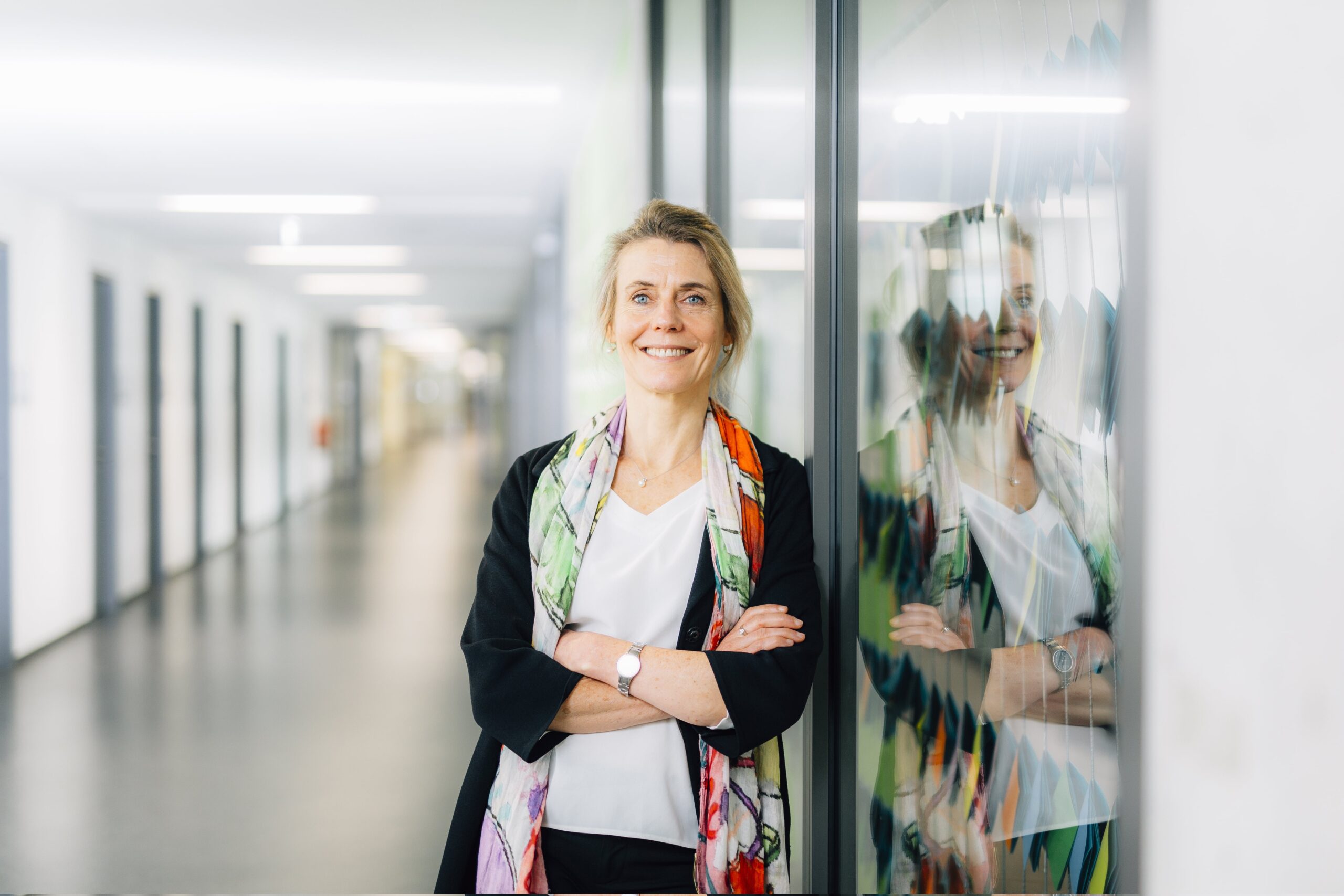
© Bielefeld University/Michael Adamski
Although this will not provide users with insight into the complete decision-making process, it will enable them to understand the AI’s recommendation without having to fully grasp how it works. ‘We are employing approaches from co-construction for this in which the aim is not only to exchange explanations with a machine as partner, but also for the machine to give proper answers as to how these explanations came about,’ says Elena Esposito.
‘Such explainable AI would be useful in many fields—not only for banks but also, for example, for insurance companies, the police, medical personnel, and a host of other areas in society,’ says Esposito. The researchers in the project are conducting pure research on how such explanations can be translated into a neutral language. Although they are also looking at existing systems, they ultimately want to develop a completely new system. What is important is for it to adapt to users and their requirements. For example, it should be able use certain signals and indications to deduce the context.
The researchers plan to start by developing a system that can be used in radiology. The answers to the same question could then differ, for example, according to whether it has been posed by medical professionals or nursing staff. Answers might also depend on the source of the question and whether there has been communication with that person in the past. This generates meaningful explanations and avoids repetition in the answers. ‘What people want to know can vary widely,’ says Philipp Cimiano.
Artificial intelligence as an adviser
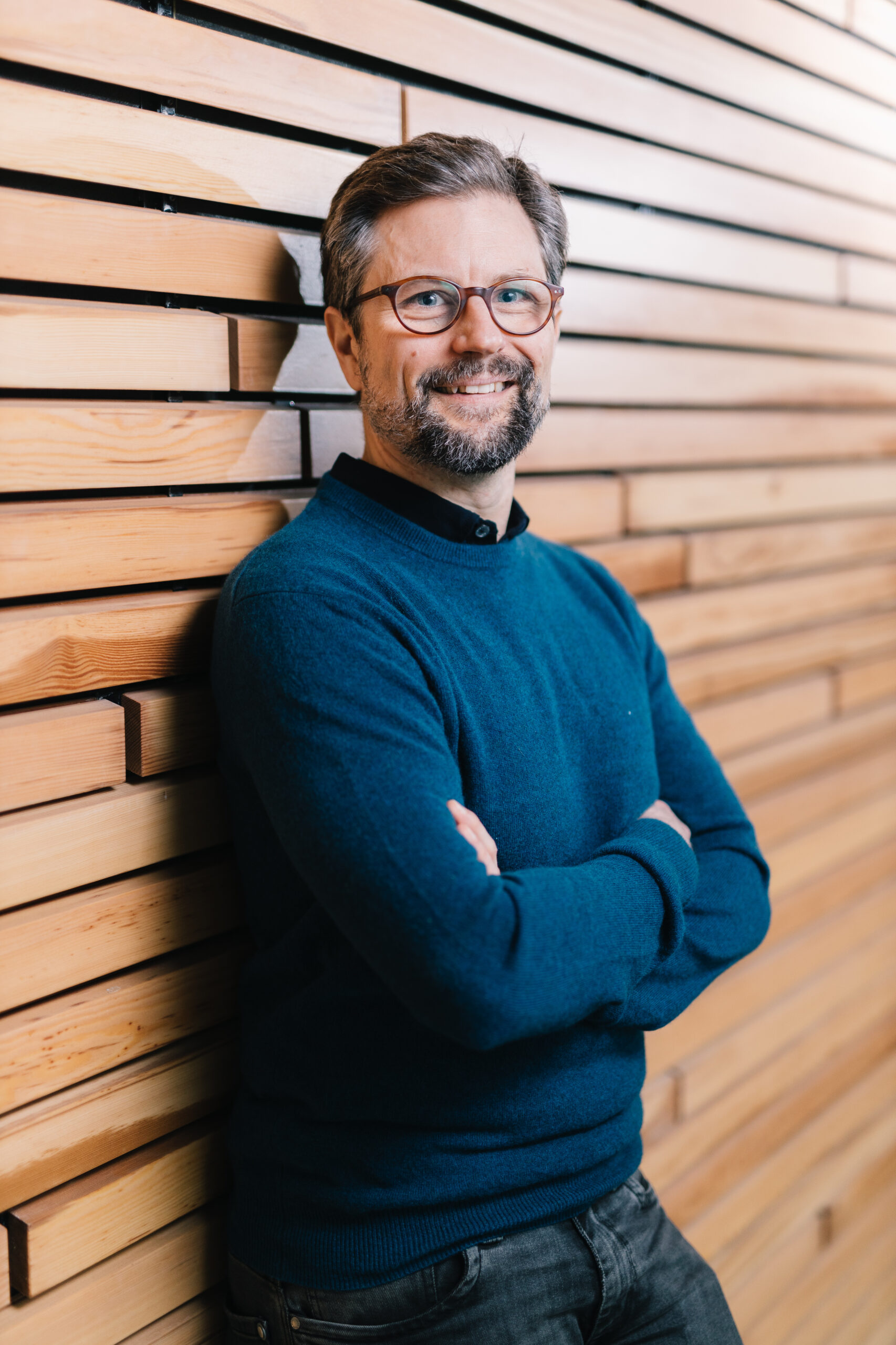
© Bielefeld University/Michael Adamski
In cooperation with the Clinic for Paediatric Surgery and Paediatric and Adolescent Urology of the Evangelisches Klinikum Bethel, the project’s researchers want to train their system using X-ray images. ‘Then we shall analyse the trial protocol and look at what kind of information users need,’ says Cimiano. Physicians would then be able to ask the system to mark the brain region that is relevant for the answer, for example. ‘They could also ask if there are any images of similar tumours that have received the same treatment. Ultimately, the focus will be on justifying a proposal for treatment and explaining it in a way that makes sense.’
In the long term, systems designed to explain decisions could not only play a role in AI applications, but also be used in robots. ‘Robots use a wide variety of models to make predictions and they classify all kinds of situations,’ says Cimiano. With robots, a dialogue system would have to be adapted to their special conditions. ‘Unlike chatbots, they move around the room in a situation,’ he says. ‘In order to do this, they not only need to contextualize, but also be able to evaluate what kind of information is relevant and how deep their explanations need to go.’