Computer vision enables self-driving vehicles to understand the environment by analysing visual data. But what if the system discovers something unknown or novel? Dr Petra Bevandić and Dr Robin Chan are focusing on these cases. The computer vision researchers are both funded by the KI-Starter programme of the state government of North Rhine-Westphalia. In the machine learning group of Professor Barbara Hammer they equip artificial intelligence (AI) models with the ability to recognize unfamiliar objects.
What is the problem you are dealing with in your research?
Robin Chan: In the field of automated driving, you need to have a perception of the environment in order to navigate safely through traffic. One problem is that most of the algorithms used are trained on a fixed set of classes. For automated driving, a popular dataset consists of 19 classes, which are roads, cars, pedestrians and so on. If you want to use the algorithms in the real world, you have to deal with its diversity, and 19 classes are not enough. But it’s difficult to add more classes because of the complexity and data requirements.
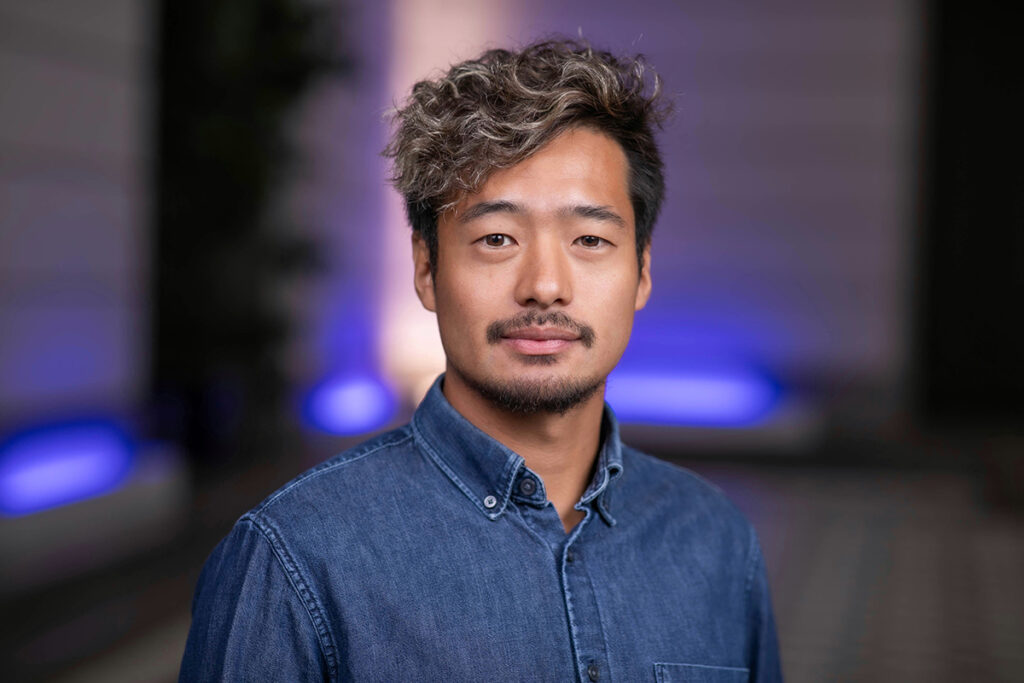
© Christian Kielmann
How do you approach this challenge?
Petra Bevandić: With my project, I want to exploit as many datasets as possible for autonomous driving training. A data set is a collection of images that are labelled according to your needs. In case of road driving you would label images from traffic scenes. Still, even if the data sets cover the same broad domain, you may find that each has a different labelling strategy. Someone looks at a road and sees a road, a car or some kind of plant. Someone else might look at the same landscape and sees building windows, doors or trees – they have a different focus. The challenge is to combine these multiple datasets with different focuses. Sometimes different class categorisations are used: ‘road‘ versus ‘own lane‘. I want to develop methods to automatically standardise such labels using general principles such as mutual relations, and thus make the datasets available for homogeneous training.
Robin Chan: We want to know the limits of the algorithm, what it doesn’t know. In the field of automated driving, it could be an object that looks different or an object it has never seen before – a semantically new object. If the algorithm doesn’t know it, it shouldn’t be forced to predict one of the 19 known classes and should be allowed to reject a prediction in such cases. My project aims to introduce a novel approach to semantic image segmentation, focusing on uncertainty estimation. When you think of cars in Germany, they look very different from those in Asia or Africa. Moreover, in different areas of the world other objects could appear on the road, for example animals. Petra’s and my research deals with these diversities. To at least identify that there is something that the model should be uncertain about. Ultimately it makes systems more capable of handling unexpected scenarios.
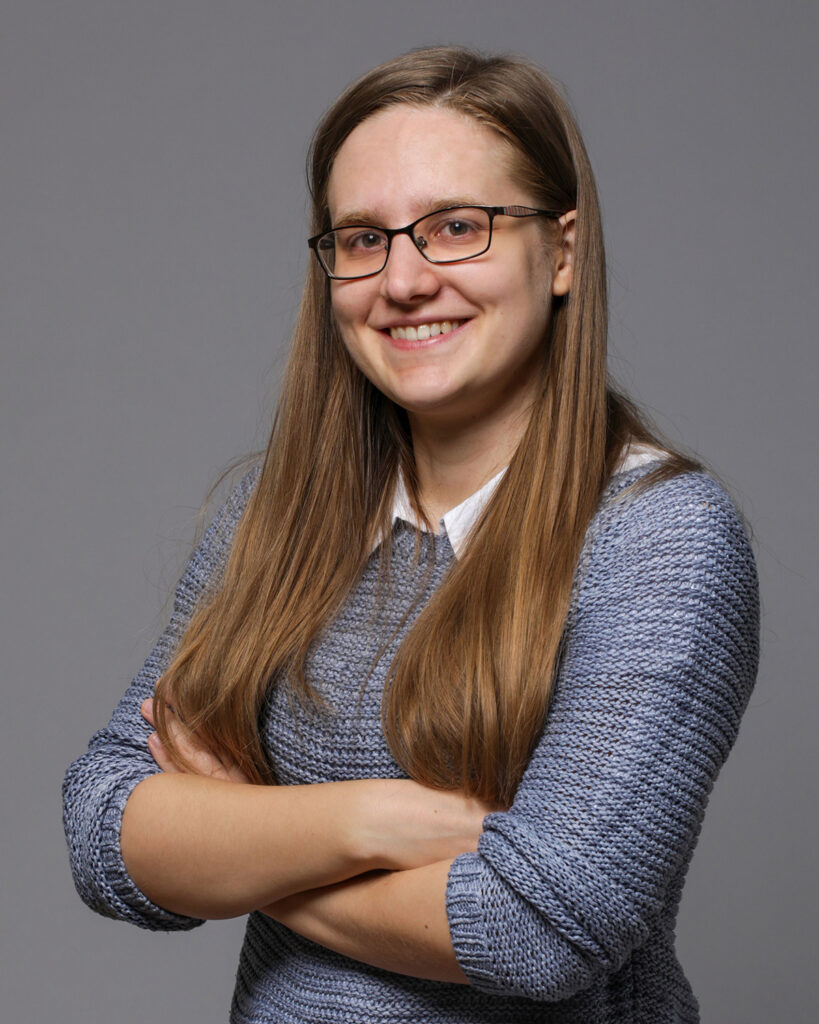
© Universität Zagreb/Saša Ćetković
Your research in Bielefeld is related to automated driving. Can your work be applied to other areas?
Robin Chan: The research is given by the datasets that are available. Automated driving is a big field that is getting a lot of attention. So, there is a lot of data to do research on. Images of roads and traffic are generated every day and are easily accessible. I have also worked with datasets to estimate the position and rotation of satellites using image data. It’s the same technology, but applied to building robots that can detect and clean up space debris. And of course, space imagery is more expensive.
Petra Bevandić: Of course, there is a wide variety of images from a wide variety of applications that can be applied in different fields. When I think about multi-data set training, I think about healthcare. One hospital has scans of patients and the staff are interested in the organs that can be seen. Another hospital focuses on the bones in the scans. How do you combine these datasets with different focuses? Can the knowledge contained in one improve the performance of our models on the other? The work is broader than automated driving.
What is the connection between your two studies? You met before the KI-Starter programme.
Petra Bevandić: I did my PhD on outlier detection. As Robin said: Can a network detect that an object is not one of the 19 classes it has learned? My approach to this question was to artificially insert non-driving objects into a driving scene. This led to the multi-dataset approach. Working on a data set focused on road driving, I wondered if I could leverage data sets with different focus to improve the performance of my road driving model. Robin’s former research group set up an outlier detection benchmark, a challenge for researchers, and my group submitted to the challenge.
How are you connected to the DataNinja research training group, which is also funded by the KI-Starter programme and aims to make AI more robust and reliable?
Robin Chan: In our machine learning group, we collaborate closely with DataNinja doctoral students whom we also supervise. In addition, we regularly participate in DataNinja events to stay connected. Making AI trustworthy is the goal of our common research. For this, it is also our responsibility to communicate what we are working on. Automated driving is a socio-technical system and will have an impact on our daily lives. Not only for car passengers, but also for pedestrians. Would you want to cross the road when there is a self-driving car? The term AI is often misused and associated with dystopian things. Therefore, being able to communicate is essential for building public trust of the public in these systems.
How do you like working in Bielefeld?
Robin Chan: The environment within CITEC and the working group makes a difference. There are so many motivated and very good PhD students. Although they come from different fields, they are always available for a chat and give inspiration, input and good feedback. That motivates. You only come up with new ideas if you talk about them. This working group gives you lots of opportunities to do that. That is a big factor in productivity.
Petra Bevandić: To introduce myself to the group, I gave a presentation about what I do. Immediately after, people came up to me and gave me feedback and ideas, even though they work in a different field. There is a genuine interest in the work of others and a sincere desire for cooperation.